What Is Incremental View Maintenance (IVM)?
Incremental View Maintenance (IVM) provides a method for keeping materialized views current by calculating and applying only incremental changes.
Join the DZone community and get the full member experience.
Join For FreeIncremental View Maintenance (IVM) provides a method for keeping materialized views current by calculating and applying only the incremental changes, as opposed to the complete recomputation of contents performed by the REFRESH MATERIALIZED VIEW command.
Materialized View in PostgreSQL
A materialized view is a database object that stores the result set of a query as a physical table, persisting the computed data for improved query performance. In contrast to regular views, materialized views contain actual data rather than merely defining a query.
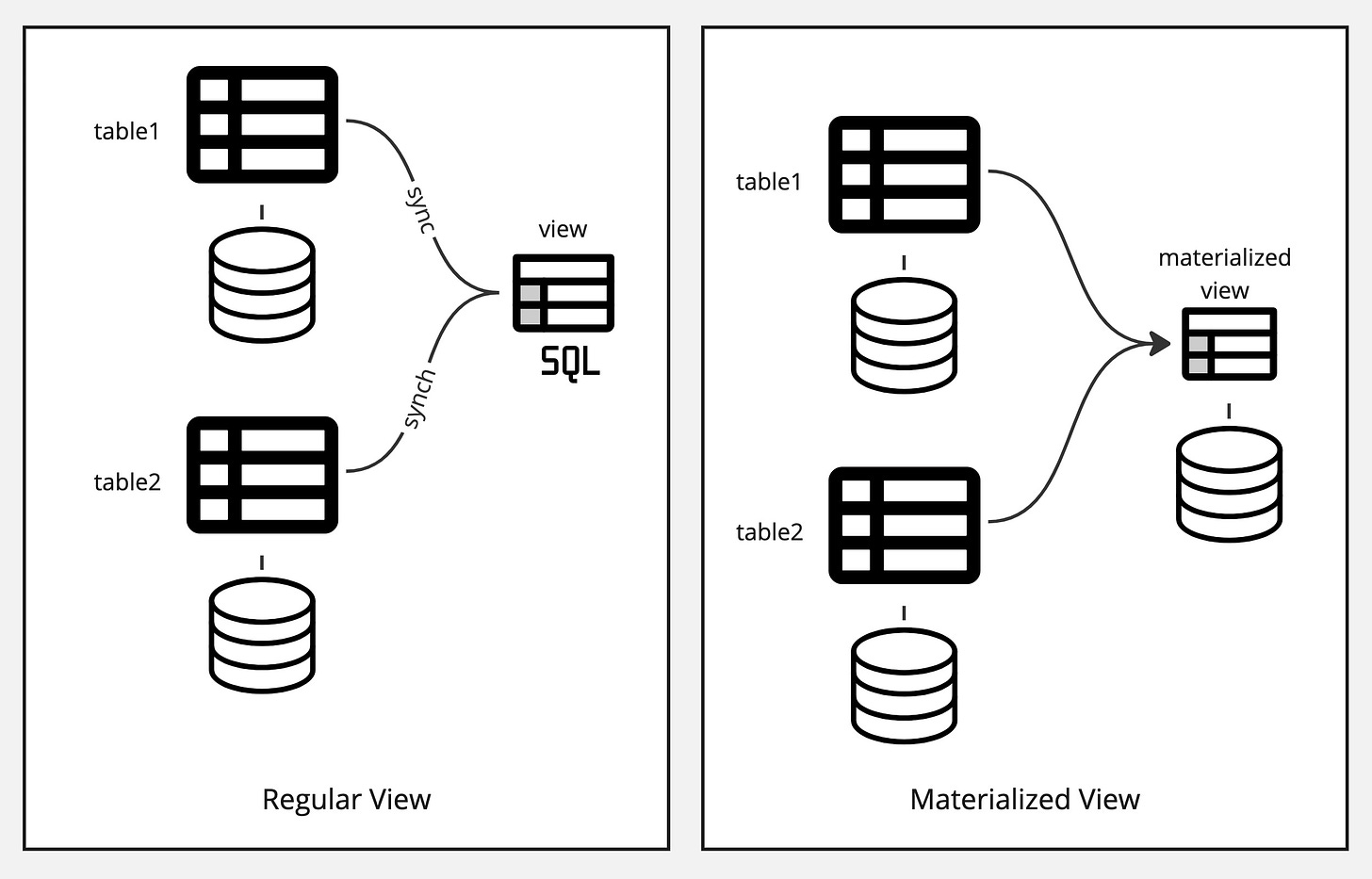
These views are advantageous for complex queries or aggregations involving large datasets, as they reduce computational overhead by storing precomputed results. Materialized views contribute to faster data retrieval, optimize specific queries, and support offline access, making them valuable in scenarios such as data warehousing, business intelligence, and decision support systems.
In PostgreSQL, you can create a materialized view using this syntax. For example:
create materialized view rental_customer as
select
r.*,
c.first_name,
c.last_name
from
customer c
join rental r on c.customer_id = r.customer_id;
What you get is a table that is enriched with a customer’s first and last names.
dvdrental=# \d rental_customer;
Materialized view "public.rental_customer"
Column | Type |
--------------+-----------------------------+
rental_id | integer |
rental_date | timestamp without time zone |
inventory_id | integer |
customer_id | smallint |
return_date | timestamp without time zone |
staff_id | smallint |
last_update | timestamp without time zone |
first_name | character varying(45) |
last_name | character varying(45) |
If we modify the customer or rental table, the materialized view is NOT updated with the latest changes. You must execute a refresh command to force the materialized view to recompute the entire dataset.
REFRESH MATERIALIZED VIEW rental_customer;
Two obvious disadvantages with materialized views in Postgres:
- It needs to be manually updated. Queries against it may not be fresh.
- When the REFRESH is invoked, the entire dataset needs to be reprocessed.
These disadvantages limit materialized views from real-time use cases. IVM can help by providing a method for keeping materialized views current.
PG_IVM Postgres Extension
IVM isn’t a feature that comes with Postgres. It’s instead available as a Postgres extension called pg_ivm. This extension can be cloned from here. Build the project and install it in Postgres with this command.
CREATE EXTENSION pg_ivm;
-- Create the IVM and select from the materialized view at the same time
select * from create_immv(
'customer_count',
'select count(*) from customer'
);
ALTER TABLE public.customer_count REPLICA IDENTITY DEFAULT;
insert into customer (store_id, first_name, last_name, address_id, active) values( 1, 'foo', 'bar', 5, 1);
-- see updated materialized view
select * from customer_count;
(You can read more about replica identity in Postgres here.)
Automatic maintenance features ensure that materialized views stay up-to-date with changes in the underlying data. pg_ivm does this incrementally.
Incremental updates refer to a method of modifying or refreshing data in a system by making only the necessary changes or additions rather than recomputing and updating the entire dataset. This approach is particularly valuable in scenarios where the overall dataset is large and frequent updates occur. Instead of processing and applying changes to the entire dataset, incremental updates identify and apply only the modifications that have occurred since the last update. This targeted updating process minimizes computational resources and reduces the time required to maintain data consistency.
Despite IVM’s benefits, considerations such as storage space and update latency must be weighed when using IVM materialized views in a database application. You may need a second Postgres that can be scaled separately from the primary instance. Alternatively, you can enable the view and consume the results externally.
Change Data Capture (CDC)
We can take our original materialized view that enriches rentals with customer information and instead create an IVM using pg_ivm and make it available via CDC.
select * create_immv('rental_customer','select
r.*,
c.first_name,
c.last_name
from
rental r
join customer c on c.customer_id = r.customer_id');
ALTER TABLE public.rental_customer REPLICA IDENTITY DEFAULT;
-- Needed if you're using Airbyte
CREATE PUBLICATION airbyte_publication FOR ALL TABLES;
Don’t forget to set the REPLICA IDENTITY.
This will allow CDC solutions like the Debezium server, Striim, or Airbyte to capture changes to the IVM materialized view and deliver it to an analytical system like Apache Pinot with UPSERT capabilities.
Flink Postgres CDC Connector
You can now capture the enriched Rental data via CDC using Ververica’s Postgres CDC connector (download here).
CREATE TABLE pgrental_customer (
rental_id int,
rental_date timestamp(3),
inventory_id int,
customer_id int,
return_date timestamp(3),
staff_id int,
last_update timestamp(3),
first_name string,
last_name string
) WITH (
'connector' = 'postgres-cdc', -- postgres cdc connector
'hostname' = 'localhost',
'port' = '5432',
'username' = 'postgres',
'password' = 'postgres',
'database-name' = 'dvdrental',
'schema-name' = 'public',
'table-name' = 'rental_customer',
'slot.name' = 'pgrental_customer',
'decoding.plugin.name'='pgoutput'
);
Does IVM Make Postgres A Streaming Database?
Not completely.
One main difference is streaming databases have the ability to consume streams from a streaming platform like Kafka and represent them as tables. Another difference is the streaming database’s ability to process events and align them by time before performing a join or aggregation, all while maintaining consistency. In IVM, time is implied, which is much easier to reason with.
Published at DZone with permission of hubert dulay. See the original article here.
Opinions expressed by DZone contributors are their own.
Comments