Usually, the first step of a data analysis consists of obtaining the data and loading the data into our work environment. We can easily download data using the following Python capability:
import urllib2
url = 'http://aima.cs.berkeley.edu/data/iris.csv'
u = urllib2.urlopen(url)
localFile = open('iris.csv'', 'w')
localFile.write(u.read())
localFile.close()
In the snippet above we used the library urllib2 to access a file on the website of the University of Berkley and saved it to the disk using the methods of the File object provided by the standard library. The file contains the iris dataset, which is a multivariate dataset that consists of 50 samples from each of three species of Iris flowers (Iris setosa, Iris virginica and Iris versicolor). Each sample has four features (or variables) that are the length and the width of sepal and petal, in centimeters.
The dataset is stored in the CSV (comma separated values) format. It is convenient to parse the CSV file and store the information that it contains using a more appropriate data structure. The dataset has 5 rows. The first 4 rows contain the values of the features while the last row represents the class of the samples. The CSV can be easily parsed using the function genfromtxt of the numpy library:
from numpy import genfromtxt, zeros
# read the first 4 columns
data = genfromtxt('iris.csv',delimiter=',',usecols=(0,1,2,3))
# read the fifth column
target = genfromtxt('iris.csv',delimiter=',',usecols=(4),dtype=str)
In the example above we created a matrix with the features and a vector that contains the classes. We can confirm the size of our dataset looking at the shape of the data structures we loaded:
print data.shape
(150, 4)
print target.shape
(150,)
We can also know how many classes we have and their names:
print set(target) # build a collection of unique elements
set(['setosa', 'versicolor', 'virginica'])
An important task when working with new data is to try to understand what information the data contains and how it is structured. Visualization helps us explore this information graphically in such a way to gain understanding and insight into the data.
Using the plotting capabilities of the pylab library (which is an interface to matplotlib) we can build a bi-dimensional scatter plot which enables us to analyze two dimensions of the dataset plotting the values of a feature against the values of another one:
from pylab import plot, show
plot(data[target=='setosa',0],data[target=='setosa',2],'bo')
plot(data[target=='versicolor',0],data[target=='versicolor',2],'ro')
plot(data[target=='virginica',0],data[target=='virginica',2],'go')
show()
This snippet uses the first and the third dimension (sepal length and sepal width) and the result is shown in the following figure:
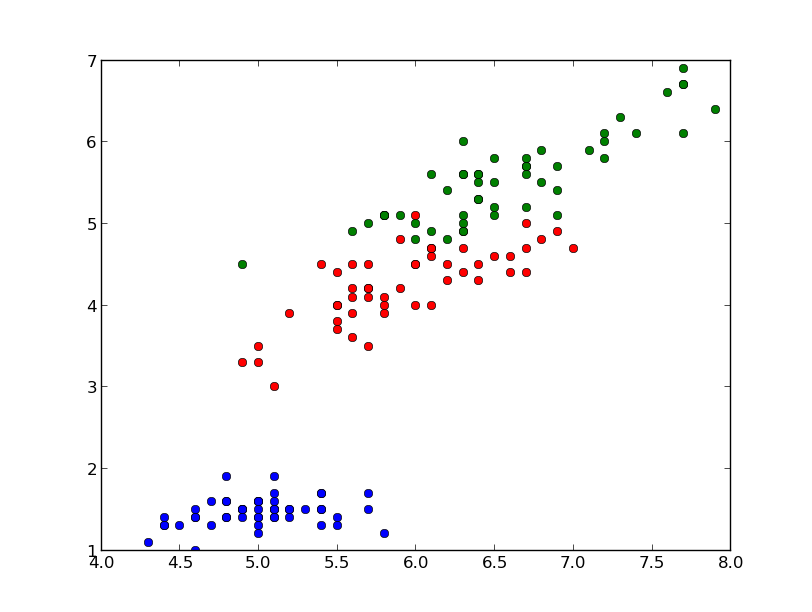
In the graph we have 150 points and their color represents the class; the blue points represent the samples that belong to the specie setosa, the red ones represent versicolor and the green ones represent virginica.
Another common way to look at data is to plot the histogram of the single features. In this case, since the data is divided into three classes, we can compare the distributions of the feature we are examining for each class. With the following code we can plot the distribution of the first feature of our data (sepal length) for each class:
from pylab import figure, subplot, hist, xlim, show
xmin = min(data[:,0])
xmax = max(data[:,0])
figure()
subplot(411) # distribution of the setosa class (1st, on the top)
hist(data[target=='setosa',0],color='b',alpha=.7)
xlim(xmin,xmax)
subplot(412) # distribution of the versicolor class (2nd)
hist(data[target=='versicolor',0],color='r',alpha=.7)
xlim(xmin,xmax)
subplot(413) # distribution of the virginica class (3rd)
hist(data[target=='virginica',0],color='g',alpha=.7)
xlim(xmin,xmax)
subplot(414) # global histogram (4th, on the bottom)
hist(data[:,0],color='y',alpha=.7)
xlim(xmin,xmax)
show()
The result should be as follows:
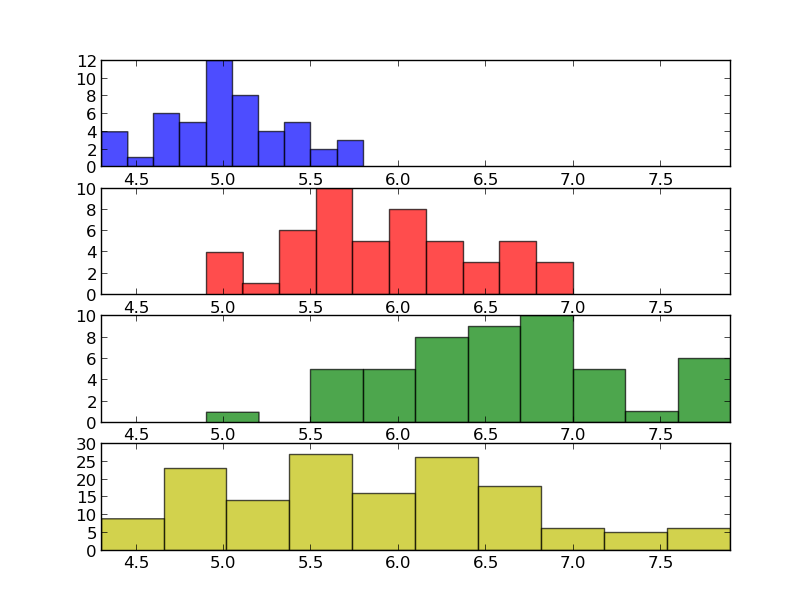
Looking at the histograms above we can understand some characteristics that could help us to tell apart the data according to the classes we have. For example, we can observe that, on average, the Iris setosa flowers have a smaller sepal length compared to the Iris virginica.