Feature Engineering for Deep Learning
Many DL neural networks contain hard-coded data processing, along with feature extraction and engineering. They may require less of these than other ML algorithms, but they still require *some*.
Join the DZone community and get the full member experience.
Join For FreeFeature engineering and feature extraction are key — and time-consuming — parts of the machine learning workflow. They are about transforming training data and augmenting it with additional features in order to make machine learning algorithms more effective. Deep learning is changing that, according to its promoters. With deep learning, one can start with raw data, as features will be automatically created by the neural network when it learns. For instance, see this excerpt from Deep Learning and Feature Engineering:
The feature engineering approach was the dominant approach till recently, when deep learning techniques started demonstrating recognition performance better than the carefully crafted feature detectors. Deep learning shifts the burden of feature design also to the underlying learning system along with classification learning typical of earlier multiple layer neural network learning. From this perspective, a deep learning system is a fully trainable system beginning from raw input, for example image pixels, to the final output of recognized objects.
As usual with bold statements, this is both true and false. In the case of image recognition, it is true that lots of feature extraction became obsolete with deep learning. Same for natural language processing where the use of recurrent neural networks made a lot of feature engineering obsolete too. No one can challenge that.
But this does not mean that data preprocessing, feature extraction, and feature engineering are totally irrelevant when one uses deep learning.
Let me take an example for the sake of clarity, taken from recommending music on Spotify with deep learning. I recommend reading this article as it introduces deep learning and how it is used in a particular case pretty well.
I will not explain what deep learning is in general. Suffice to say that deep learning is most often implemented via a multi-layer neural network. An example of a neural network is given in the above article:
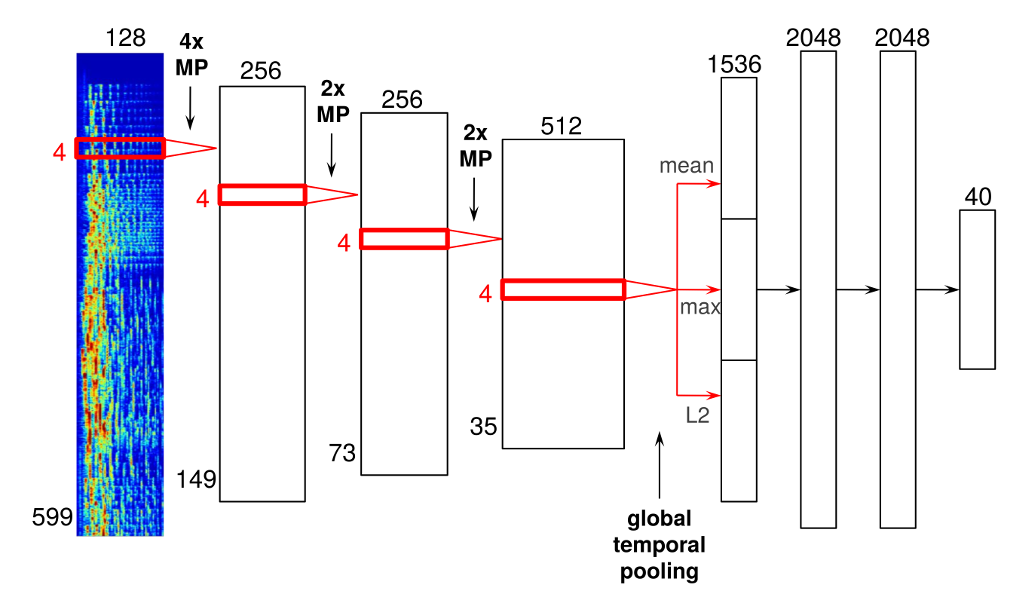
Data flows from left to right. The input layer, the leftmost layer, receives an encoding of songs. The next three layers are max-pooling layers. The next layer computes mean, max, and L2 norm of its input data. The next three layers are convolutional layers, and the last layer is a temporal pooling layer.
Don't worry if you don't fully understand what all this means. The key point is that learning only happens between the three convolutional layers. All the other layers are hard-coded feature extraction and hard-coded feature engineering. Let me explain why:
- The input data is not raw sound data. The input data is a spectrogram representation of the sound obtained via a Fourier transform. That data transformation happens outside the neural network. This is a drastic departure from the above quote that claims deep learning starts with raw data.
- The next three levels are max pooling. They rescale their input data to smaller dimension data. This is hard-coded, and it is not modified by learning.
- The next level computes the mean, the max, and the L2 norm of time series. This is a typical feature engineering step. Again, this is hard-coded and not modified by learning.
- The next three levels are convolutional levels. Learning happens on the connections between the first two convolutional levels and on the connections between the last two convolutional levels.
- The last level computes statistics on the data output by the last convolutional level. This is also a typical feature engineering. It is also hard-coded and not modified by learning.
This example is a neural network where most of the network is some hard-coded feature engineering or some hard-coded feature extraction. I write hard-coded as these are not learned by the system, they are predefined by the network designer, the author of the article. When that network learns, it adjusts weights between its convolutional layers, but it does not modify the other arcs in the network. Learning only happens for 2 pairs of layers, while our neural network has seven pairs of consecutive layers.
This example is not an exception. The need for data preprocessing and feature engineering to improve the performance of deep learning is not uncommon. Even for image recognition, where the first deep learning success happened, data preprocessing can be useful. For instance, finding the right color space to use can be very important. Max pooling is also used a lot in image recognition networks.
The conclusion is simple: Many deep learning neural networks contain hard-coded data processing, feature extraction, and feature engineering. They may require less of these than other machine learning algorithms, but they still require some.
I am not the only one stating the above; see this article, for instance.
Note: The need for data pre-processing for deep learning is getting more attention. Google just announced tf.Transform to address that need for TensorFlow users.
Published at DZone with permission of Jean Francois Puget, DZone MVB. See the original article here.
Opinions expressed by DZone contributors are their own.
Comments