The Outbox Pattern
Learn how the outbox pattern ensures reliable message delivery across distributed systems, safeguarding and forwarding diverse data types effectively.
Join the DZone community and get the full member experience.
Join For FreeIn the intricate tapestry of distributed systems, particularly within the microservices architecture, the challenges of reliable message delivery and data consistency are pervasive and demanding. The outbox pattern, often cited in the exclusive realm of microservices, emerges from a crucible where ensuring the atomicity of local database transactions and publishing messages to other services is paramount. Amidst the web of inter-service communication, it emerges as a stratagem to ensure that messages are not forgotten in the midst of outages or network inconsistencies.
However, the adaptability and utility of the outbox pattern transcends its microservices origins, weaving into its fabric principles that are universally applicable to diverse architectural contexts. This paper embarks on a journey to demystify the outbox pattern, not as a microservices-specific tool, but as a robust architectural pattern with the potential to improve reliability and consistency across disparate and distributed computing environments.
While the outbox pattern initially sprouted from the complexities of microservices, ensuring stable message exchanges amidst potential pitfalls, it’s clear that its benefits are not confined to this singular architectural style. Simply put, the outbox pattern is about catching, securely holding, and reliably sending messages, addressing a widespread challenge familiar to architects across various technological landscapes, especially in scenarios where dependable messaging is crucial.
In this exploration, we aim to break down the outbox pattern, shedding light on its foundational mechanics and principles, all with the goal of helping architects grasp its benefits and thoughtfully apply them in various contexts beyond its initial birthplace. By diving into where the pattern originated and explaining its key features, we hope to pave a way for architects to adeptly use the outbox pattern, leveraging its abilities to bolster reliability and consistency across varied architectural scenarios.
What Is the Outbox Pattern?
Embarking upon the exploration of the outbox pattern, one discerns not merely a technique for reliable message delivery but a universally applicable principle capable of bridging diverse use cases and varied inputs. Whether it be event messages, data packets, or other forms of communication in distributed systems, the outbox pattern emerges not just as a courier but as a meticulous steward, safeguarding and ensuring the successful journey of diverse data forms from sender to recipient through its pivotal phases: intercept, store, and chain.
Intercept
Within the intercept phase, the outbox pattern unpretentiously embraces diverse types of data — be it events, messages, or other digital communiqués — pausing them before their onward journey. Here, any piece of data poised for transmission is intercepted, underscoring the pattern’s generic and adaptable nature. The essence is not bound to a specific data type or message format, but rather it stands as a universally applicable pause, ensuring that no piece of transmitted data is hurriedly lost in the convolution of transmission.
Store
Segueing into the store phase, the outbox provides an unbiased, secure residence for the intercepted data. It does not discriminate against the type or nature of the data it safeguards; whether messages, events, or otherwise, every piece of data is enveloped in a protective haven, guarding against premature loss and ensuring that they are poised for reliable future transmission. This universal store underscores the pattern’s versatility, ready to house varied data types until they are sturdily dispatched onward.
Chain
In the final chain phase, data —regardless of its type or origin — is diligently and reliably conveyed from the outbox to its destination. It transcends the mere act of forwarding messages; it’s a commitment to ensuring the consummation of data transmission of varied natures. The data’s creation and its delivery are decoupled, allowing for tailored management of each, ensuring that every byte, every message, and every event, however constituted, is undeterred in its journey, even amidst potential disruptions.
Through these lenses, the outbox pattern unveils itself not merely as a conduit for event messages but as a universally applicable principle, ensuring the safe and reliable transit of varied data types across distributed landscapes. As we dissect its mechanics, the aim is to illuminate the adaptable nature of this pattern, inspiring architects to envisage its applications across myriad contexts and diverse data types, ensuring reliability, and consistency in the broad tapestry of digital communication.
This could be a representation of an outbox pattern:
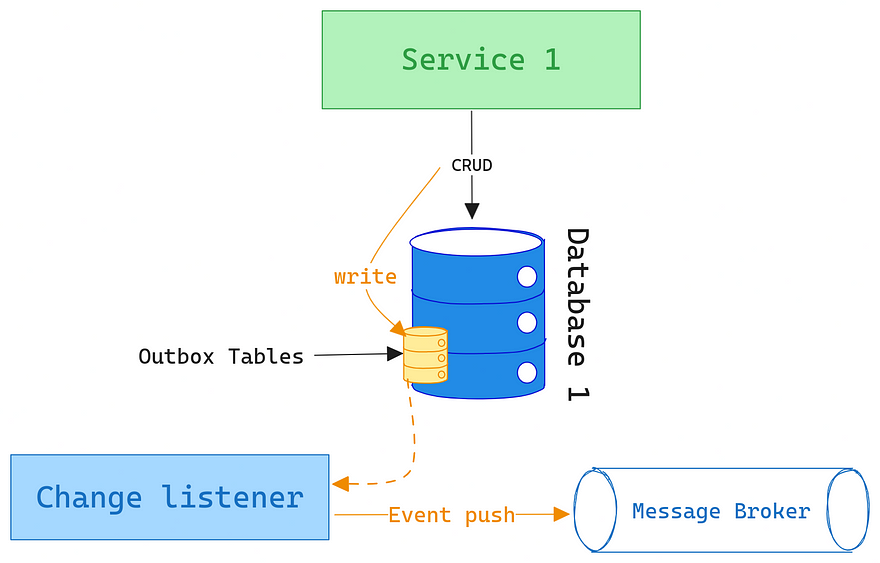
Outbox and Inbox
In the vast expanse of distributed systems, the outbox pattern diligently safeguards the journey of a message, ensuring its reliable dispatch, while its mirror counterpart, the inbox pattern, manages its controlled reception and handling upon arrival at its destination. When a message is securely dispatched through the outbox, the inbox adroitly assumes control, safeguarding incoming data and ensuring that, even amidst multiple receipts due to network inconsistencies, messages are processed just once, thereby maintaining data consistency and idempotent handling. Together, the outbox and inbox patterns forge a harmonious and synchronized data flow, crafting a robust communication framework that assures seamless and consistent data transmission and reception across distributed systems.
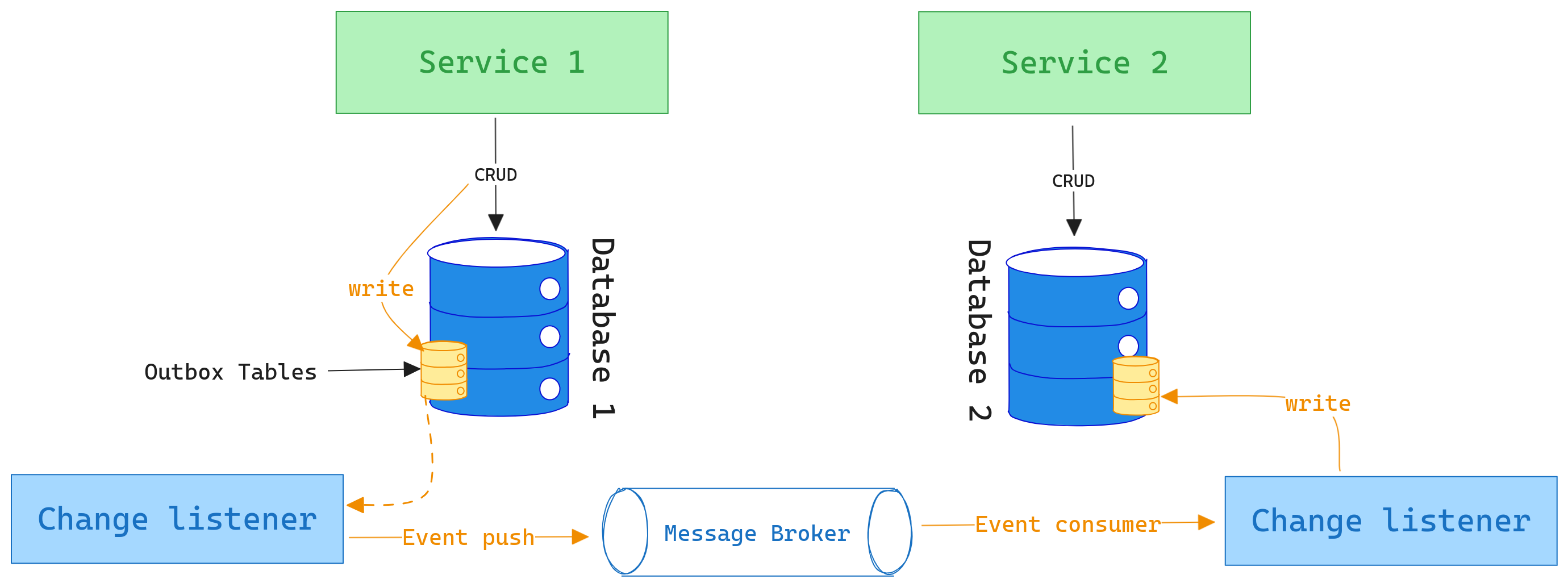
Make the Difference With Change Data Capture (CDC)
Change data capture is fundamentally about capturing and tracking changes in a dataset. Whenever data changes (be it through creation, updates, or deletions), CDC mechanisms ensure that these alterations are identified and made available for other systems to consume. CDC can work on a polling basis, where consumers frequently check for alterations, or a push basis, where changes are forwarded to consumers as they occur.
It could be represented as follows:
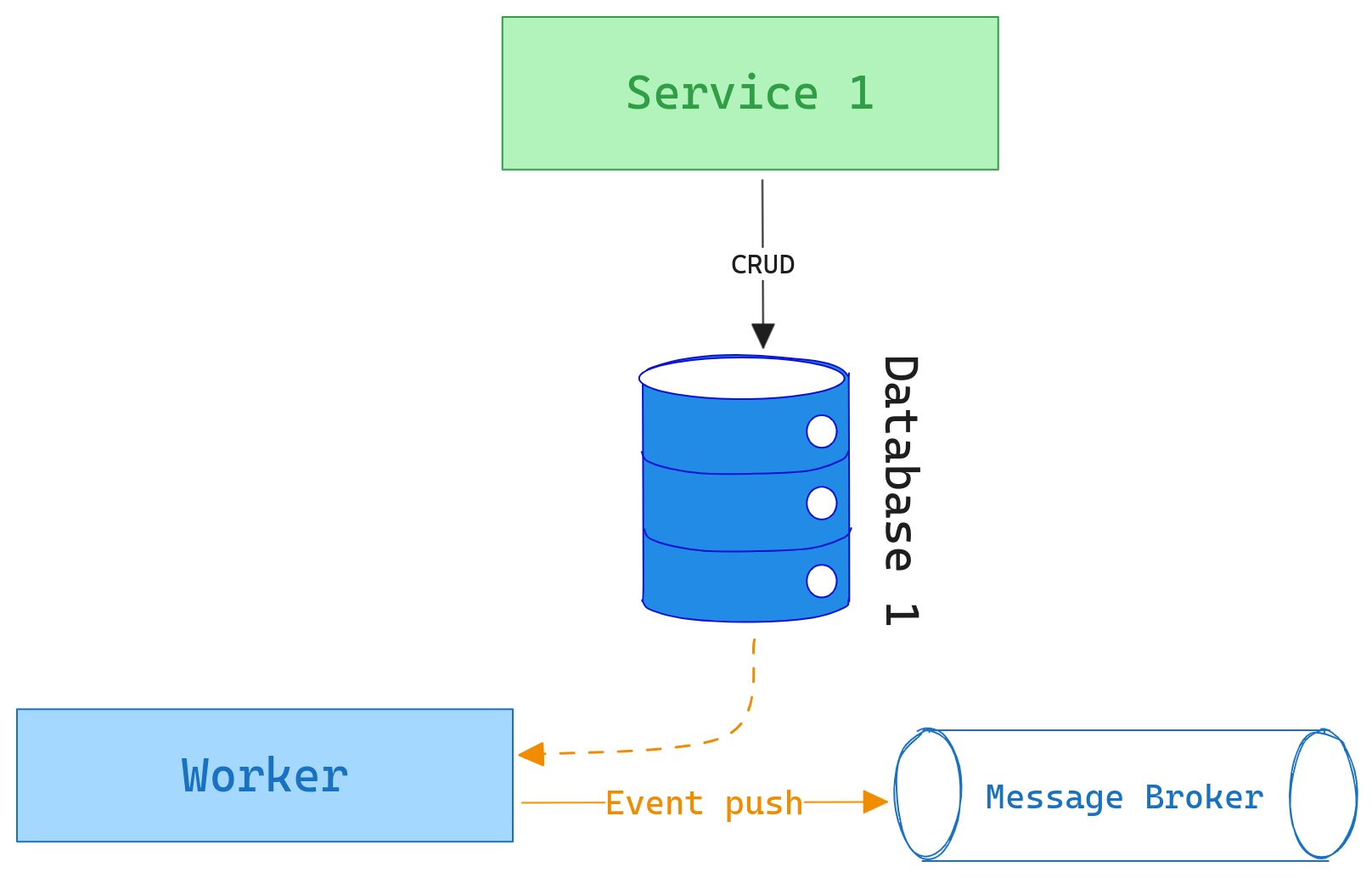
The outbox pattern, as described earlier, doesn’t focus on capturing every change in the data. Instead, it seeks to create a reliable intermediary for events or messages destined for other services or components. The outbox acts as a temporary storage, ensuring messages are not lost during transient failures and providing a layer of insulation between message creation and delivery.
Benefits and Trade-Offs
In the odyssey of digital communications within distributed systems, the outbox pattern emerges as a beacon, safeguarding data transmission with its astute principles. As we delve into its merits and demerits, it’s crucial to perceive them not as absolute, but as considerations to be harmoniously balanced by architects, tailoring its application to varied contexts beyond its microservices origin.
Benefits
Enhanced Reliability
A fundamental merit of the Outbox Pattern is its robustness against data loss during transmission failures. By intercepting and storing messages pre-transmission, it ensures that data is not lost amidst potential disruptions or system failures.
Decoupling of Concerns
The separation between data creation and delivery, achieved through the intercept-store-chain trifecta, allows systems to manage each aspect independently. This decoupling fosters an environment where alterations or disruptions in one domain do not cascade into the other.
Versatility in Data Handling
The generic adaptability of the outbox pattern, welcoming varied data types and message formats, enables it to be a versatile tool, allowing architects to deploy it across numerous scenarios, ensuring data consistency and reliable message delivery irrespective of the context.
Coordinated Communication With Inbox
In tandem with the inbox pattern, the outbox ensures a harmonious data flow, offering a symmetrically balanced architecture where messages are not only securely sent but also controlled and managed efficiently upon reception.
Trade-Offs
Implementation Complexity
The meticulous weaving of intercept, store, and chain phases necessitates an additional layer of architectural complexity. Ensuring seamless operation, particularly in systems not originally designed with the outbox in mind, can be a daunting task.
Latency in Message Delivery
By introducing an intermediary store-and-forward mechanism, messages might experience a delay from creation to delivery, which might be a vital consideration in scenarios demanding real-time communication.
Resource Utilization
The necessity to store messages, even transiently, demands additional resources both in terms of storage and computational overhead. For systems with stringent resource constraints, this utilization needs to be judiciously considered.
Management of Failures
While the outbox safeguards against message loss, the management, and mitigation of failures — ensuring messages eventually reach their destination post-failure — warrants additional strategies and could introduce additional complexities.
Conclusion
By exploring the benefits and trade-offs of the outbox pattern, architects are armed with a nuanced understanding that enables them to apply the pattern judiciously where its merits shine, while mitigating or accepting its inherent complexities and costs. Whether in the midst of the microservices from which it was born, or in new, diverse architectural landscapes, the outbox pattern stands ready to improve data reliability and consistency, provided its trade-offs are navigated with astuteness and foresight.
Published at DZone with permission of Pier-Jean MALANDRINO. See the original article here.
Opinions expressed by DZone contributors are their own.
Comments