Better explaining the CAP Theorem
Join the DZone community and get the full member experience.
Join For Free
today, i thought a lot about how to examine different databases. choosing a database is often a daunting task. there's a lot of confusion, a 'theorem', and more than all, the immortal proverb 'not one size fits all'. as if it helps.
- consistency: every read would get you the most recent write
- availability: every node (if not failed) always executes queries
- partition-tolerance: even if the connections between nodes are down, the other two (a & c) promises, are kept.
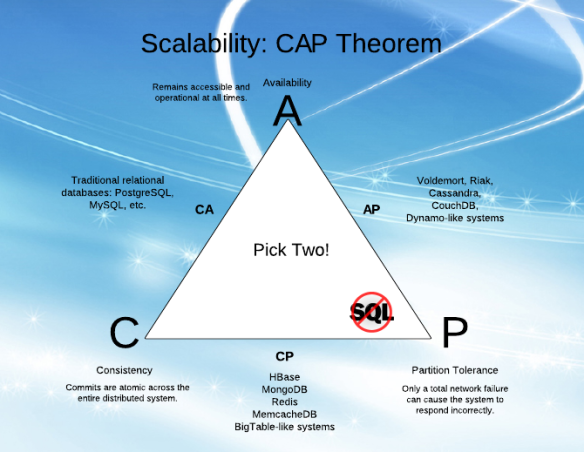
it's really just a vs c!
- availability is achieved by replicating the data across different machines
- consistency is achieved by updating several nodes before allowing further reads
- total partitioning, meaning failure of part of the system is rare. however, we could look at a delay, a latency, of the update between nodes, as a temporary partitioning . it will then cause a temporary decision between a and c:
- on systems that allow reads before updating all the nodes, we will get high availability
- on systems that lock all the nodes before allowing reads, we will get consistency
by the way, there's no distributed system that wants to live with "paritioning" - if it does, it's not distributed. that is why putting sql in this triangle may lead to confusion.
Opinions expressed by DZone contributors are their own.
Comments