Maximizing AI Training Efficiency: Selecting the Right Model
Unlock AI training efficiency: Learn to select the right model architecture for your task. Explore CNNs, RNNs, Transformers, and more to maximize performance.
Join the DZone community and get the full member experience.
Join For FreeTraining models quickly and accurately is important for building trust in these workflow tools. With AI-powered applications becoming more capable of executing complex tasks, data scientists and machine learning engineers can explore new novel approaches.
To develop the best model for a specific use case, utilizing the appropriate model, dataset, and deployment can streamline the AI development process and yield the best results.
Selecting the Right Model
Selecting the best model architecture is important for getting the best results for your specific task. Different kinds of problems need different model architectures:
- Convolutional Neural Networks (CNNs)
- Recurrent Neural Networks (RNNs)
- Transformer models
- GANs and Diffusion Models
- Reinforcement Learning
- Autoencoders
When choosing a model architecture, think about things like the kind of data you have, how complicated your task is, and the resources you have. It's often a good idea to start with simpler models and make them more complicated as needed. Apart from the 6 listed, there are other models you can explore.
1. Convolutional Neural Network (CNNs)
CNNs are ideal for image processing tasks and excel in extracting patterns like edges, textures, and objects within visual data by using filters that detect spatial relationships.
- Use cases: Image classification, object detection
- Compute requirements: High GPU compute requirements since visual processing is GPU-intensive
- Notable architectures: EfficientNet, ResNet, CNNs with attention mechanisms
Convolutional Neural Networks have been around for quite some time now, using weights and parameters to evaluate, classify, and detect objects in computer vision models. With the surge of the transformer architecture, ViTs or Vision Transformers have also become a strong alternative.
2. Recurrent Neural Networks (RNNs)
RNNs are best suited for sequential or time-dependent data, where the order of information is crucial. They're widely used in applications like language modeling, speech recognition, and time-series forecasting, as RNNs can retain previous input states, making them effective for capturing dependencies within sequences.
- Use cases: Sequential data, time series analysis, speech recognition, forecasting
- Compute requirements: Moderate to high GPU compute
- Notable architectures: LSTM, GRU, bidirectional RNNs
RNNs previously were designed to power natural language processing tasks but have been superseded by Transformer models like BERT and GPT. However, RNNs remain relevant for highly sequential tasks and real-time analysis such as weather modeling and stock forecasting.
3. Transformer Models
Transformer models revolutionized AI for sequence data, especially in natural language processing tasks. Transformers process entire text sequences in parallel, using self-attention to weigh the importance of different tokens, words, and phrases in context. This parallelism boosts their performance on complex language-based tasks. Transformers do suffer if training is not properly tuned, trained on quality data, or not trained enough resulting in hallucinations or false positives.
- Use cases: Language processing, text generation, chatbots, knowledge base
- Compute requirements: Training requires extreme GPU compute and running requires moderate to high GPU compute
- Popular architectures: BERT and GPT
Transformer models can be augmented since they are prompted. Thus, Mixture of Experts and Retrieval Augmented Generation are methods for enhancing the functionality of a highly generalized AI model.
4. Image Generation Models: Diffusion and GANs
Diffusion and GANs are for generating new, realistic images. These image-generation models are popular in creative fields for generating images, videos, or music, and they're also used for data augmentation in training models.
- Use cases: Image generation by prompt, image augmentation, artistic ideation, 3D model generation, image upscaling, denoising
- Compute requirements: GANs can be parallelized whereas diffusion models are sequential. Both require high GPU requirements, especially for higher fidelity generation.
- Popular architectures: StableDiffusion, Midjourney, StyleGAN, DCGAN
Diffusion models utilize denoising and image recognition techniques to guide the model in generating a persuasive image. Hundreds of passes will make static fuzz into an original art piece.
GANs or general adversarial networks pit two competing models in an iterative dance: one generator for creating an image and a discriminator for evaluating if the generated image is fake or real. Continuous passes will train both models to become better and better until the generator is able to beat the discriminator.
5. Reinforcement Learning
Reinforcement Learning (RL) is well-suited for decision-making tasks that involve interacting with an environment to achieve a specific objective. RL models learn by trial and error, making them ideal for applications in robotics, game-playing, and autonomous systems, where the model receives feedback from its actions to progressively improve its performance. RL shines in scenarios where the AI must develop strategies over time, balancing short-term actions with long-term goals.
- Use cases: Gameplay optimization, exploit finding, creating competitive CPUs, decision-making
- Compute requirements: Depends on complexity, but benefits with more GPU compute
- Popular architectures: Q-Learning, DQN, SAC
You can find various instances of hobbyists creating RL-based AIs to train how to play a game. Tuning and training of a reinforcement learning model requires reading in-between lines so as to not allow the AI to learn an unintended action. For example, in a driving game Trackmania, the AI practitioner did not allow the AI the ability to brake, encouraging speed when taking a turn. He did not want the AI to learn how to take a turn successfully if it meant constant braking.
6. Autoencoders
Autoencoders are a type of unsupervised neural network designed to learn efficient codings of input data by compressing it into a lower-dimensional representation and then reconstructing it. This involves an encoder compressing the input and a decoder reconstructing it. Autoencoders are particularly well-suited for tasks such as dimensionality reduction, data denoising, and anomaly detection. They excel in applications like image and signal processing, where they can remove noise from data or detect unusual patterns that deviate from the norm. Additionally, they are used in generating synthetic data and feature extraction, making them versatile tools in various machine learning and data preprocessing tasks.
- Use cases: Data compression, anomaly detection, and noise reduction
- Compute requirements: Moderate compute; can run on mid-range GPUs for smaller data
- Popular architectures: Vanilla Autoencoders, Variational Autoencoders (VAE)
Model Selection Guidelines
We developed a table and a rough flow chart to help push you in the right direction in choosing the appropriate AI model for your use case. These are just suggestions and there are numerous other models to pick from but this can get you started.
Model | Use Case | GPU Compute Requirement |
---|---|---|
Convolutional Neural Network | Image Processing, Classification, and Detection | ⭐⭐⭐⭐ |
Recurrent Neural Network | Sequential Data, Time Series | ⭐⭐⭐ |
Transformers | Complex Natural Language, Chatbots, Knowledge Bases | ⭐⭐⭐⭐⭐ |
General Adversarial Networks | Data Generation | ⭐⭐⭐⭐ |
Diffusion Models | Image Generation | ⭐⭐⭐⭐ |
Reinforcement Learning | Decision Making, Robotics, Games | ⭐⭐⭐ |
Autoencoders | Data Compression, Anomaly Detection | ⭐⭐⭐ |
Model Selection Decision Tree
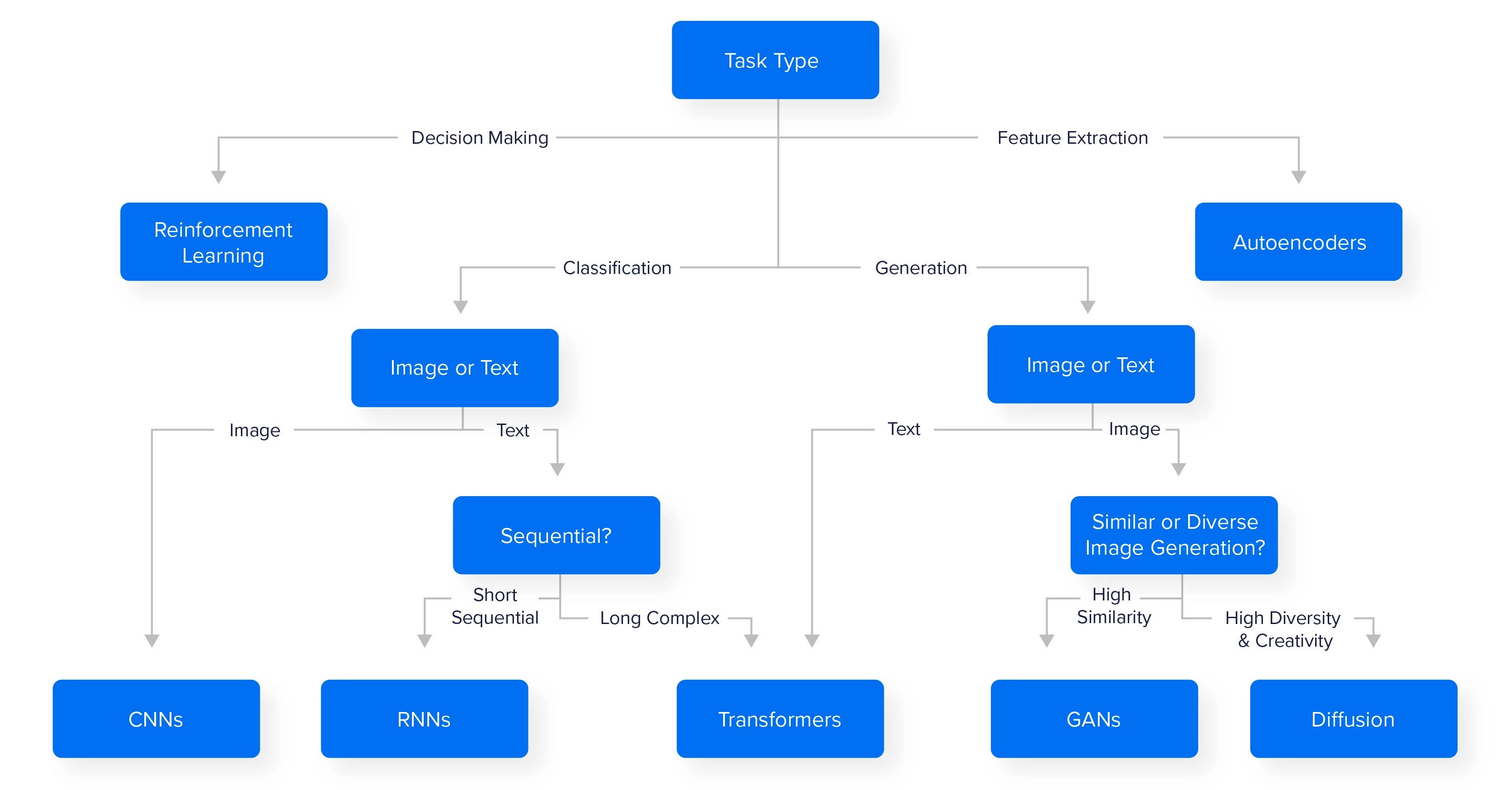
There are also alternatives to CNNs that utilize transformers called ViTs as well as other models that may perform better for your specific use case. We encourage practitioners to experiment with different architectures to achieve the desired result.
But to train these models efficiently, run exploratory analysis, and benchmark various codes is not computationally cheap. High-performance hardware is necessary for faster train time completion.
Published at DZone with permission of Kevin Vu. See the original article here.
Opinions expressed by DZone contributors are their own.
Comments