RAG From a Beginner to Advanced: Introduction [Video]
By integrating RAG, we can overcome many limitations of traditional LLMs, providing more accurate, up-to-date, and domain-specific answers.
Join the DZone community and get the full member experience.
Join For FreeIn this blog post, we’ll explore:
- Problems with traditional LLMs
- What is Retrieval-Augmented Generation (RAG)?
- How RAG works
- Real-world implementations of RAG
Problems With Traditional LLMs
While LLMS have revolutionized the way we interact with technology, they come with some significant limitations:
Hallucination
LLMs sometimes hallucinate, meaning they provide factually incorrect answers. This occurs because they generate responses based on patterns in the data they were trained on, not always on verified facts.
- Example: An AI might state that a historical event occurred in a year when it didn’t.
Outdated Knowledge
Models like GPT-4 have a knowledge cutoff date (e.g., May 2024). They lack information on events or developments that occurred after this date.
- Implication: The AI cannot provide insights on recent advancements, news, or data.
Untraceable Reasoning
LLMs often provide answers without clear sources, leading to untraceable reasoning.
- Transparency: Users don’t know where the information came from.
- Bias: The training data may contain biases, affecting the output.
- Accountability: Difficult to verify the accuracy of the response
Lack of Domain-Specific Expertise
While LLMs are good at generating general responses, they often lack domain-specific expertise.
- Outcome: Answers may be generic and not delve deep into specialized topics.
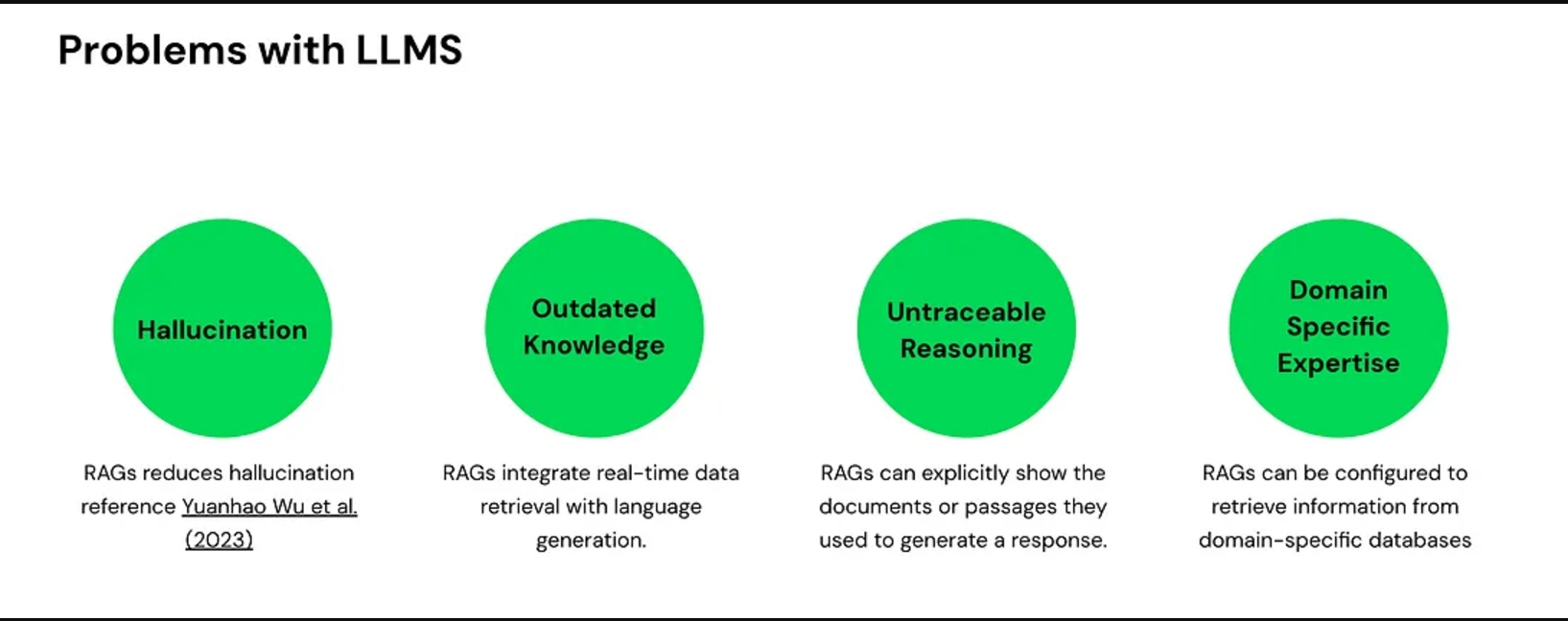
Problems with LLM
What Is RAG?
Imagine RAG as your personal assistant who can memorize thousands of pages of documents. You can later query this assistant to extract any information you need.
RAG stands for Retrieval-Augmented Generation, where:
- Retrieval: Fetches information from a database
- Augmentation: Combines the retrieved information with the user’s prompt
- Generation: Produces the final answer using an LLM
How RAG Works: The Traditional Method vs. RAG
Traditional LLM Approach
- A user asks a question.
- The LLM generates an answer based solely on its trained knowledge base.
- If the question is outside its knowledge base, it may provide incorrect or generic answers.
RAG Approach
1. Document Ingestion
- A document is broken down into smaller chunks.
- These chunks are converted into embeddings (vector representations).
- The embeddings are indexed and stored in a vector database.
2. Query Processing
- The user asks a question.
- The question is converted into an embedding using the same model.
- A search engine queries the vector database to find the most relevant chunks.
- The top relevant results are retrieved.
3. Answer Generation
- The retrieved information and the user’s question are combined.
- This combined input is passed to the LLM (like GPT-4 or LLaMA).
- The LLM generates a context-aware answer.
- The answer is returned to the user.
Real-World Implementations of RAG
General Knowledge Retrieval
- Input extensive documents (hundreds or thousands of pages)
- Efficiently extract specific information when needed
Customer Support
- RAG-powered chatbots can access real-time customer data.
- Provide accurate and personalized responses in sectors like finance, banking, or telecom
- Improved first-response rates lead to higher customer satisfaction and loyalty
Legal Sector
- Assist in contract analysis, e-discoveries, or regulatory compliances
- Streamline legal research and document review processes
Video
Conclusion
As Thomas Edison once said:
“Vision without execution is hallucination.”
In the context of AI:
“LLMs without RAG are hallucination.”
By integrating RAG, we can overcome many limitations of traditional LLMs, providing more accurate, up-to-date, and domain-specific answers.
In upcoming posts, we’ll explore more advanced topics on RAG and how to obtain even more relevant responses from it. Stay tuned!
Thank you for reading!
Published at DZone with permission of Mohammed Talib. See the original article here.
Opinions expressed by DZone contributors are their own.
Comments